The focus on risk is essential for AI trading in stocks to succeed, particularly in high-risk markets. Here are 10 top tips to incorporate effective risk management practices in your AI trading strategies:
1. Define Risk Tolerance
Tip: Clearly establish the maximum acceptable loss for each trade, daily drawdowns, and the overall portfolio losses.
What's more, understanding your risk threshold will help you establish specific guidelines to your AI trading systems.
2. Automated stop-loss and take-profit orders
Tip Use AI to dynamically implement and adjust stop-loss/take-profit levels based on volatility.
Why? Automated security measures limit losses and lock-in profits without emotional involvement.
3. Diversify Your Portfolio
Diversify your investment into different sectors, assets and markets.
Why: Diversification helps to make sure that potential gains and losses are balanced by reducing the risk associated with each asset.
4. Set Position Sizing Rules
Make use of AI to calculate positions sizes on the following criteria:
Portfolio size.
Risk per trade (e.g. 1 to 2 percent of the total portfolio value).
Asset volatility.
The reason: Proper sizing of your position prevents overexposure to high-risk trades.
5. Be aware of volatility and modify your strategies accordingly.
Tip: Assess the market's volatility frequently using indicators such as VIX (stocks), or even on-chain (copyright).
Why: Higher volatility requires tighter risk controls and adaptive trading strategies.
6. Backtest Risk Management Rules
Tips Include risk-management parameters, such as stop loss levels, as well as position sizing, in backtests to assess their efficacy.
The reason: Testing is essential to ensure that your risk measures work in different market conditions.
7. Implement Risk-Reward Ratios
Tips: Ensure that each trade has an appropriate ratio between risk and reward, like 1:3 (risking $1 to make $3).
The reason: Consistently using favorable ratios improves long-term profitability even in the event of occasional losses.
8. AI that detects and responds to anomalies
Create an anomaly detection program to identify unusual trading patterns.
The early detection of a market allows the possibility of removing positions or alter strategies prior to a significant move in the market.
9. Hedging Strategies to Incorporate
Tips: Make use of hedging strategies such as futures or options to reduce the risk.
Penny stocks are hedges with ETFs for the sector, or other securities that are related to the sector.
copyright: Use stablecoins to hedge your portfolio or inverted exchange-traded funds.
Why should you take a risk to hedge against price swings?
10. Regularly monitor and adjust risk parameters
Tip: As the market changes, review and update your AI system's risk settings.
Why? Dynamic risk management lets you adjust your strategy to different market situations.
Bonus: Use Risk Assessment Metrics
Tip: Evaluate your strategy using metrics like:
Maximum drawdown: largest portfolio loss between trough and peak.
Sharpe Ratio: Risk-adjusted return.
Win-Loss Ratio: Number of profitable trades relative to losses.
What are they? They offer insight into your strategy's performance and risk exposure.
You can increase your AI trading techniques' effectiveness and security by using these tips. Check out the recommended stocks ai hints for website tips including ai trading app, best ai for stock trading, ai predictor, ai trading bot, best copyright prediction site, best stock analysis app, copyright ai trading, best ai trading app, coincheckup, best ai stock trading bot free and more.
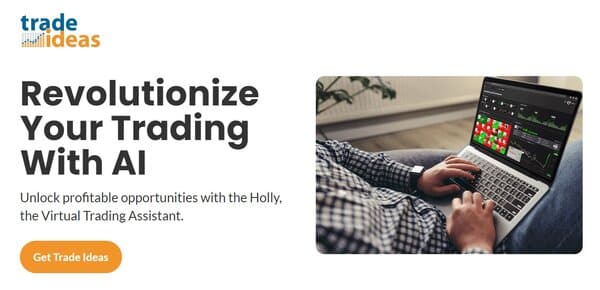
Top 10 Tips To Leveraging Ai Backtesting Software For Stock Pickers And Predictions
Backtesting is a powerful tool that can be used to improve AI stock strategy, investment strategies, and forecasts. Backtesting gives insight into the performance of an AI-driven strategy in the past in relation to market conditions. Here are 10 top tips to backtesting AI tools to stock pickers.
1. Use high-quality historic data
Tip: Ensure that the software used for backtesting is precise and up-to date historical data. These include stock prices and trading volumes as well dividends, earnings reports, and macroeconomic indicators.
What's the reason? Good data permits backtesting to reflect market conditions that are realistic. Incorrect or incomplete data could result in false backtests, which can affect the reliability and accuracy of your strategy.
2. Include Realistic Trading Costs and Slippage
Tip: Simulate realistic trading costs such as commissions as well as transaction fees, slippage and market impact during the process of backtesting.
What's the reason? Not taking slippage into consideration can cause your AI model to overestimate its potential returns. Including these factors ensures your backtest results are closer to real-world trading scenarios.
3. Test Different Market Conditions
TIP: back-testing your AI Stock picker to multiple market conditions like bear markets or bull markets. Also, include periods of volatility (e.g. an economic crisis or market corrections).
Why AI-based models might behave differently depending on the market environment. Tests under different conditions will make sure that your strategy can be flexible and able to handle different market cycles.
4. Test with Walk-Forward
Tips: Conduct walk-forward tests. This lets you test the model against an unchanging sample of historical data before confirming its accuracy using data from outside of your sample.
What is the reason? Walk-forward tests can help assess the predictive powers of AI models based on unseen evidence. This is a more accurate measure of the performance of AI models in real-world conditions than static backtesting.
5. Ensure Proper Overfitting Prevention
Tip: Avoid overfitting the model by testing it with different time periods and ensuring it doesn't pick up noise or anomalies from old data.
What causes this? It is because the model is focused on historical data. As a result, it's not as effective in predicting market movement in the near future. A balanced model can adapt to different market conditions.
6. Optimize Parameters During Backtesting
Tip: Backtesting is a excellent method to improve important variables, such as moving averages, positions sizes and stop-loss limit, by repeatedly adjusting these parameters and evaluating the impact on returns.
The reason: Optimizing the parameters can improve AI model efficiency. As previously mentioned, it's crucial to ensure that the optimization does not result in an overfitting.
7. Drawdown Analysis and Risk Management: Integrate Both
TIP: Consider the risk management tools, such as stop-losses (loss limits), risk-to reward ratios, and position sizing when testing the strategy back to gauge its strength against massive drawdowns.
The reason: a well-designed risk management strategy is vital to long-term financial success. Through simulating how your AI model does when it comes to risk, it's possible to identify weaknesses and adjust the strategies to provide better risk adjusted returns.
8. Examine Key Metrics Other Than Returns
You should focus on other indicators than simple returns such as Sharpe ratios, maximum drawdowns winning/loss rates, as well as volatility.
These metrics help you understand the risk-adjusted return of your AI strategy. If you focus only on the returns, you might overlook periods with high risk or volatility.
9. Simulate Different Asset Classes & Strategies
Tips: Try testing the AI model using different asset classes (e.g. stocks, ETFs and copyright) in addition to different investing strategies (e.g. mean-reversion, momentum or value investing).
Why is it important to diversify a backtest across asset classes can help evaluate the adaptability and performance of an AI model.
10. Regularly Update and Refine Your Backtesting Approach
Tip. Update your backtesting with the most up-to-date market data. This ensures that it is current and is a reflection of changing market conditions.
Why: The market is dynamic as should your backtesting. Regular updates ensure that the results of your backtest are relevant and that the AI model continues to be effective even as new information or market shifts occur.
Make use of Monte Carlo simulations to determine the risk
Tip: Monte Carlo Simulations are excellent for modeling many possible outcomes. It is possible to run several simulations, each with a different input scenario.
Why? Monte Carlo simulations are a fantastic way to determine the probability of a range of scenarios. They also give an in-depth understanding of risk particularly in volatile markets.
Backtesting is a great way to improve the performance of your AI stock-picker. Backtesting is an excellent method to ensure that AI-driven strategies are trustworthy and flexible, allowing you to make better decisions in volatile and ebbing markets. Follow the top rated additional resources about trading bots for stocks for blog recommendations including ai day trading, best stock analysis app, trading chart ai, best ai penny stocks, ai investing, stock trading ai, ai trading platform, ai stock trading app, trading ai, coincheckup and more.
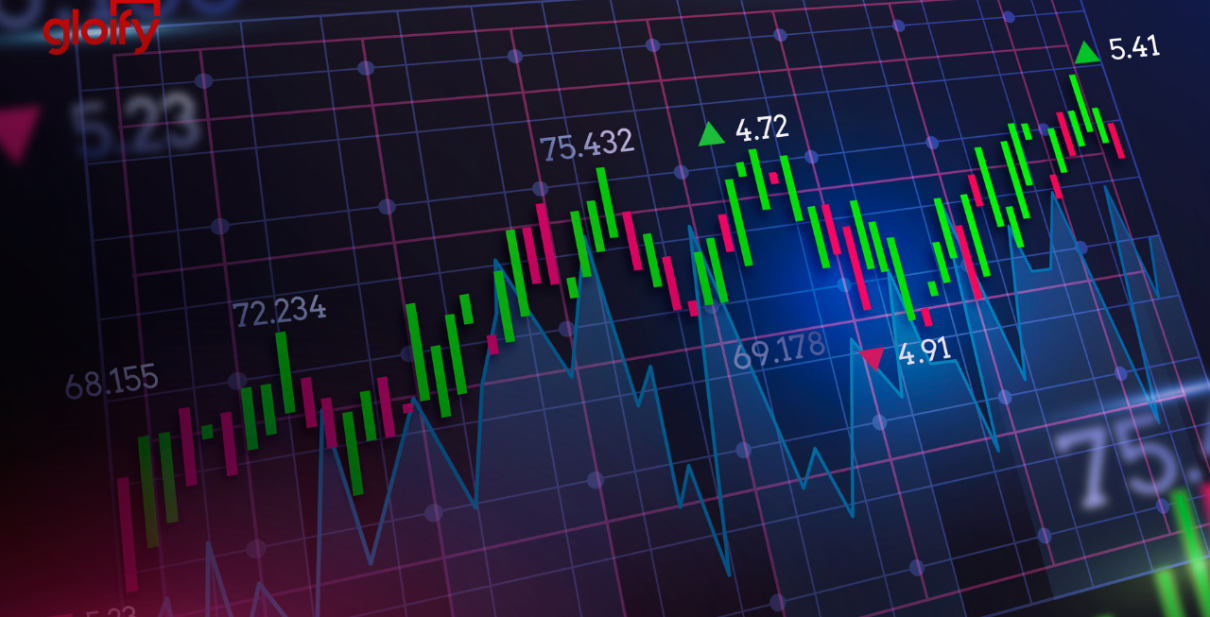